As multi-modal transport solutions are adopted in cities across the UK, junctions and the systems that control them need to develop, writes David Watts, ITS engineer for Transport for Greater Manchester (TfGM)…
The coronavirus pandemic has highlighted more than ever the need for transport authorities to adapt to changes to the transport network, in ways that existing systems are perhaps unable to.
Engineers require more dynamic tools and systems to better respond to changing conditions and multi-modal users. Through the use of artificial intelligence (AI) and new technologies, some of TfGM’s recent work on smart junctions has highlighted the possibilities of what the future of signal control may look like.
TfGM currently operates over 1,250 adaptive traffic signal control systems (1,000 SCOOT sites and 250 MOVA sites), providing significant benefits to the region’s 10 district councils by responding to changing levels of demand and reducing vehicle delay. However, the technologies need to evolve to meet wider transport management demands.
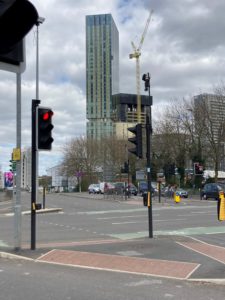
SCOOT – which stands for Split Cycle Offset Optimisation Technique – detects vehicles as they approach a signalised junction well in advance of the stop line. This detection, from multiple junctions, is fed into a central system, which models the flow of traffic in the area.
MOVA, or Microprocessor Optimised Vehicle Actuation, is similar to SCOOT in that it is a method of controlling the traffic signals based on the presence of vehicles detected on the approach to a signalised junction, but it controls one junction at a time rather than looking at traffic flow as a whole.
However, a weakness of these adaptive traffic signal control systems is the inability to incorporate pedestrians and cyclists into their algorithms.
The technologies do exist to capture their volumes in real time, but there are concerns that the data provided isn’t accurate enough to develop widespread cycle- and pedestrian-friendly adaptive control.
Political will and appetite have also contributed to historically maintaining a vehicle-first approach to traffic signal control, though attitudes are starting to change in recent years. The pandemic, coupled with the government’s ambitious carbon reduction targets, have increased the need for greater provision for cyclists and pedestrians across the nation’s highway networks to encourage people out of their cars.
Developments in intelligent transport systems in recent years, such as the use of floating vehicle data and video analytics have provided a much more granular level of data to local authorities, allowing for greater use of data in decision making. TfGM has made significant strides in this respect and can utilise an ever-growing repertoire of data sources to better understand travel behaviour across the region.
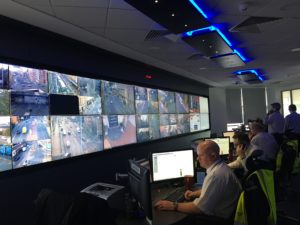
This has prompted research and development into new approaches to traffic signal control that can take advantage of this improved data, such as the Smart Junctions project. As part of this, over 100 AI sensors have been installed across over 13 junctions and two PED crossings, providing much greater insight into all modes of transport across an area in Salford and Manchester city centre.
Working closely with Vivacity, TfGM installed several of its on-street control units, enabling an algorithm or ‘agent’ to take control of traffic signal timings. Vivacity was able to adopt an AI approach to optimisation using reinforcement learning (RL) where the agent learns from millions of hours of experience in a simulation prior to deployment.
Training the agent involves initially allowing it to choose random traffic stages and then, by using the inputs from the sensors (such as positions, speeds, and waiting times of different road users), it can see what the traffic looked like before and after each signal stage and assess how “good” a particular action was for a given scenario. Through iteration and experimentation in the simulation, the agent learns good traffic signal control behaviour, before it is deployed in the real world.
Adopting this unique approach to signal control has demonstrated early positive results. The AI is taking sensible, realistic decisions whilst in control, serving vehicle stages with the most demand whilst ensuring pedestrians are also captured and served when appropriate. The agent has appeared to be more dynamic in terms of stage selection, particularly in the off-peak periods.
The next stage for TfGM is to allow the ‘agent’ to take control of the trial sites more frequently. This will allow it to gather more performance data and make more performance observations in comparison with current systems. Evaluation will take account of all users of the road network – pedestrians, cycles, buses, cars when considering what is success rather than the previous approach of considering only the impact on car traffic.