Researchers at Uber’s Advanced Technologies Group, the University of Toronto and UC Berkeley have developed a new technique to predict pedestrian behaviour called discrete residual flow network (DRF-NET).
According to the researchers, this neural network can make predictions about future pedestrian behaviour while capturing “the inherent uncertainty in forecasting long-range motion”.
“Our learned network effectively captures multimodal posteriors over future human motion by predicting and updating and discretising distribution over spatial locations,” the researchers wrote in a paper detailing the technique.
In it, the researchers expressed beliefs about the future positions of pedestrians through categorical distributions that represent space. They then used these distributions to plan and optimise paths for self-driving vehicles, which take into account the expected positions of pedestrians.
Firstly, the DTF-NET network introduced in their paper converts images of road maps into a picture composed of discrete pixels. The behaviours of pedestrians are thus encoded into a bird’s-eye view rasterised image, which is aligned with a detailed semantic map.
Subsequently, the network extracts features that regarded as particularly useful for predicting the behaviour of pedestrians from the rasterised images. Finally, the researchers trained their model to predict the future behaviour of pedestrians on the road based on these features.
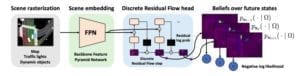
The researchers trained and evaluated their neural network using a large-scale dataset previously compiled, which contains real world recordings with object annotations and online detection-based tracks, collected in several cities in North-America.
These recordings include pedestrian trajectories that were manually annotated by the researchers in a 360-degree, 120m-range (394ft) view using an on-vehicle lidar sensor.
In the evaluations carried out by the researchers, the DTF-NET technique reportedly performed well, outperforming several other baseline methods for predicting pedestrian behaviour.
This method could thus potentially help to enhance the performance of Uber’s self-driving vehicles, allowing them to anticipate the movements of pedestrians and plan their paths accordingly.
“The strong performance of DRF-NET’s discrete predictions is very promising for cost-based and constrained robotic planning,” the researchers wrote.
The pedestrian behaviours processed and predicted by the DTF-NET network include ‘pending jaywalk’, ‘ongoing jaywalk’ and ‘sidewalk traversal’, as well as crosswalk.